Explore Maplestar: Your Ultimate Guide & More!
Is Maplestar the next big thing in predictive analytics, or just another flash in the pan? The answer, according to those who've witnessed its impact firsthand, is a resounding affirmation of its disruptive potential.
Maplestar, a name increasingly whispered in hushed tones within boardrooms and tech conferences alike, represents a paradigm shift in how organizations understand and leverage data. It's not merely about crunching numbers; it's about unlocking actionable insights that drive strategic decision-making, optimize operational efficiency, and ultimately, fuel sustainable growth. The core strength of Maplestar lies in its ability to synthesize vast, disparate datasets from market trends and customer behavior to internal performance metrics and identify patterns that would otherwise remain hidden. This capability, powered by advanced algorithms and machine learning models, empowers businesses to anticipate future outcomes, mitigate risks, and seize emerging opportunities with unprecedented agility.
The buzz surrounding Maplestar isn't just hype. Several high-profile companies across diverse sectors have already adopted the platform and are reporting significant gains. A leading e-commerce retailer, for example, used Maplestar to predict seasonal demand fluctuations with remarkable accuracy, allowing them to optimize inventory levels and minimize stockouts. A major financial institution leveraged the technology to identify fraudulent transactions in real-time, preventing substantial financial losses. And a healthcare provider utilized Maplestar to predict patient readmission rates, enabling them to implement targeted interventions that improved patient outcomes and reduced healthcare costs. These are just a few examples of the transformative impact that Maplestar is having on organizations across the globe. But what are the key components and how does it actually work?
- Breaking Who Is Jordana Lajoie The Actress You Need To Know
- Breaking Who Is Maggie Siff The Actress You Need To Know Now
Maplestar's architecture is built on a foundation of scalable cloud infrastructure, ensuring the platform can handle even the most demanding data processing workloads. Its modular design allows organizations to customize the system to their specific needs, integrating seamlessly with existing data sources and analytics tools. The platform's user interface is intuitive and user-friendly, empowering both data scientists and business users to access and interpret insights without requiring specialized technical expertise. Moreover, Maplestar incorporates robust security measures to protect sensitive data and ensure compliance with relevant regulations. But at its heart, the real power of Maplestar lies in its proprietary algorithms and machine learning models. These sophisticated algorithms are constantly learning and adapting, refining their predictions over time and providing organizations with increasingly accurate and reliable insights. The platform also incorporates advanced feature engineering techniques, automatically identifying the most relevant variables for predicting specific outcomes. This eliminates the need for manual feature selection, saving data scientists valuable time and resources. In addition, Maplestar offers a range of advanced analytical capabilities, including predictive modeling, prescriptive analytics, and what-if scenario analysis. These tools empower businesses to not only understand what is likely to happen in the future, but also to determine the optimal course of action to achieve their desired outcomes.
While the potential benefits of Maplestar are undeniable, organizations must carefully consider several factors before implementing the platform. First, they need to ensure they have access to high-quality data. The accuracy and reliability of Maplestar's predictions are directly dependent on the quality of the data it is trained on. Second, they need to have a clear understanding of their business objectives. Maplestar is a powerful tool, but it is only effective if it is used to solve specific business problems. Third, they need to invest in the necessary training and resources to ensure their employees can effectively use the platform. Maplestar is user-friendly, but it still requires a certain level of expertise to interpret the results and translate them into actionable insights. Finally, they need to be prepared to embrace a data-driven culture. Maplestar is not a magic bullet; it is a tool that can help organizations make better decisions, but it requires a commitment to using data to guide those decisions. The adoption of Maplestar requires a fundamental shift in mindset, encouraging a culture of experimentation and continuous improvement.
The landscape of predictive analytics is rapidly evolving, with new technologies and approaches emerging constantly. Maplestar is at the forefront of this revolution, pushing the boundaries of what is possible with data analysis. However, it is important to note that Maplestar is not the only player in this space. There are several other vendors offering similar solutions, each with its own strengths and weaknesses. Organizations need to carefully evaluate their options and choose the platform that best meets their specific needs and requirements. Ultimately, the success of any predictive analytics initiative depends not only on the technology itself, but also on the people who use it and the processes that support it. Organizations need to invest in the necessary skills and resources to ensure they can effectively leverage these tools to achieve their business objectives. The future of Maplestar, and indeed the future of predictive analytics, is bright. As data volumes continue to grow and analytical techniques become more sophisticated, these technologies will play an increasingly important role in helping organizations make better decisions and achieve sustainable growth. The ongoing development of artificial intelligence and machine learning will only serve to further enhance the capabilities of platforms like Maplestar, unlocking even greater potential for data-driven innovation.
- Why Are Graciebon Videos So Popular The Secret Is Out
- Olivia Surratt More Than Just Kevin Surratt Jrs Wife Facts
The implications of Maplestar extend far beyond the realm of business. The technology has the potential to address some of the world's most pressing challenges, from climate change and disease prevention to poverty reduction and social justice. By leveraging the power of data, we can gain a deeper understanding of these complex problems and develop more effective solutions. For example, Maplestar could be used to predict the spread of infectious diseases, allowing public health officials to implement targeted interventions and prevent outbreaks. It could also be used to identify communities at risk of food insecurity, enabling aid organizations to provide timely assistance. And it could be used to detect and prevent human trafficking, helping to protect vulnerable populations. The possibilities are endless. The challenge lies in ensuring that these technologies are used responsibly and ethically, and that the benefits are shared equitably across society. This requires a commitment to transparency, accountability, and collaboration. We need to engage in open dialogue about the potential risks and benefits of these technologies, and we need to develop clear guidelines for their use. Only then can we harness the full potential of Maplestar and other predictive analytics tools to create a better future for all.
One of the key differentiators of Maplestar is its commitment to explainable AI (XAI). Many traditional machine learning models are "black boxes," making it difficult to understand why they make certain predictions. This lack of transparency can be problematic, especially in sensitive applications such as healthcare and finance. Maplestar addresses this issue by providing insights into the underlying reasoning behind its predictions. This allows users to understand why a particular outcome is predicted, and to identify potential biases or errors in the model. XAI is not just about transparency; it is also about building trust. When users understand how a model works, they are more likely to trust its predictions and to use them effectively. This can lead to better decision-making and improved outcomes. Maplestar's commitment to XAI reflects its broader commitment to responsible AI. The company recognizes that AI is a powerful tool, but it must be used in a way that is ethical, transparent, and accountable. This requires a focus on fairness, privacy, and security. Maplestar is actively working to address these challenges and to ensure that its technology is used for the benefit of society.
The future of Maplestar depends on its ability to adapt to the ever-changing landscape of data and analytics. The company is constantly investing in research and development, exploring new technologies and approaches to improve its platform. One area of focus is on the integration of natural language processing (NLP) and computer vision. NLP can be used to extract insights from unstructured text data, such as customer reviews and social media posts. Computer vision can be used to analyze images and videos, providing valuable information about consumer behavior and market trends. By combining these technologies with its existing predictive analytics capabilities, Maplestar can provide organizations with an even more comprehensive view of their business environment. Another area of focus is on the development of edge computing solutions. Edge computing involves processing data closer to the source, rather than sending it to a centralized cloud server. This can reduce latency, improve security, and enable new applications in areas such as manufacturing and transportation. Maplestar is working to develop edge computing solutions that can be deployed in a variety of environments, allowing organizations to leverage the power of predictive analytics at the edge of their network. The company's long-term vision is to create a truly intelligent platform that can anticipate the needs of its users and provide them with the right information at the right time. This requires a deep understanding of the business context and the ability to seamlessly integrate data from multiple sources. Maplestar is well-positioned to achieve this vision, thanks to its strong team, its cutting-edge technology, and its commitment to innovation.
Maplestar Overview Category Information Type Predictive Analytics Platform Key Features Machine Learning, Predictive Modeling, Data Integration, Explainable AI, Scenario Analysis Industry Applications E-commerce, Finance, Healthcare, Manufacturing, Retail, Transportation Deployment Cloud-based, On-premise (custom options) Pricing Subscription-based (tiered pricing based on usage and features) Website Official Maplestar Website (Example Website - Replace with actual URL) Data Security Compliance SOC 2, GDPR, HIPAA ready features. Explainable AI Capabilities Provides insights into the reasoning behind predictions, increasing transparency and trust.
The impact of Maplestar can also be felt in areas beyond direct business applications. For instance, government agencies are exploring the use of Maplestar to optimize resource allocation, improve public services, and enhance citizen engagement. Law enforcement agencies are using the platform to predict crime patterns and allocate resources more effectively. Educational institutions are using it to identify students at risk of dropping out and provide them with targeted support. These are just a few examples of how Maplestar is being used to make a positive impact on society. The key is to ensure that these applications are developed and deployed in a way that is ethical, transparent, and accountable. This requires a collaborative effort between technology developers, policymakers, and community stakeholders. We need to have open and honest conversations about the potential risks and benefits of these technologies, and we need to develop clear guidelines for their use. Only then can we ensure that Maplestar and other predictive analytics tools are used to create a more just and equitable society.
Consider the implications for smaller businesses. While large corporations often have the resources to invest in expensive data science teams and custom-built analytics solutions, smaller businesses may struggle to compete. Maplestar levels the playing field by providing a cost-effective and easy-to-use platform that can be accessed by businesses of all sizes. This democratizes access to advanced analytics, empowering smaller businesses to make better decisions and compete more effectively. For example, a small retail store could use Maplestar to predict customer demand for different products, allowing them to optimize inventory levels and avoid stockouts. A small restaurant could use it to predict customer traffic patterns, allowing them to optimize staffing levels and minimize wait times. A small manufacturing company could use it to predict equipment failures, allowing them to schedule preventative maintenance and avoid costly downtime. These are just a few examples of how Maplestar can help smaller businesses thrive. The key is to provide them with the necessary training and support to effectively use the platform. This requires a focus on user-friendly interfaces, comprehensive documentation, and responsive customer support. By empowering smaller businesses with the power of data, we can create a more vibrant and competitive economy.
In conclusion, Maplestar represents a significant advancement in the field of predictive analytics. Its ability to synthesize vast amounts of data, identify hidden patterns, and generate actionable insights is transforming the way organizations make decisions. While challenges remain in terms of data quality, skills gaps, and ethical considerations, the potential benefits of Maplestar are undeniable. As the technology continues to evolve and become more accessible, it is likely to play an increasingly important role in shaping the future of business and society.
The ethical dimensions of using a tool like Maplestar cannot be ignored. As predictive algorithms become more deeply integrated into decision-making processes across various sectors, concerns about bias, fairness, and accountability inevitably arise. It's crucial to acknowledge that algorithms are only as unbiased as the data they are trained on. If the training data reflects existing societal biases, the algorithm is likely to perpetuate and even amplify those biases. This can have serious consequences, particularly in areas such as criminal justice, employment, and lending. For example, a predictive policing algorithm trained on biased crime data could disproportionately target certain communities, leading to further discrimination and mistrust. Similarly, a hiring algorithm trained on biased resume data could perpetuate gender or racial inequalities in the workplace. To mitigate these risks, it's essential to carefully audit the data used to train predictive algorithms, and to implement measures to detect and correct bias. It's also important to be transparent about how these algorithms are being used, and to provide individuals with the opportunity to challenge decisions that are based on algorithmic predictions. Furthermore, accountability mechanisms need to be put in place to ensure that those who develop and deploy these algorithms are held responsible for their impact. By addressing these ethical challenges proactively, we can ensure that predictive analytics technologies like Maplestar are used to promote fairness, equity, and social justice.
The role of human oversight in the age of predictive analytics is also critical. While Maplestar and other similar platforms can automate many aspects of the decision-making process, they should not be viewed as a replacement for human judgment. Instead, they should be seen as a tool to augment human capabilities and to provide decision-makers with more information and insights. In many cases, human judgment is needed to interpret the results of predictive models, to consider factors that are not captured in the data, and to make ethical judgments. For example, a doctor might use a predictive model to assess a patient's risk of developing a certain disease, but ultimately the doctor needs to use their clinical judgment and experience to make a treatment decision that is tailored to the individual patient's needs. Similarly, a loan officer might use a predictive model to assess a borrower's creditworthiness, but the loan officer needs to consider other factors, such as the borrower's character and circumstances, before making a lending decision. Human oversight is also important for detecting and correcting errors in predictive models. No model is perfect, and even the most sophisticated algorithms can make mistakes. Human experts can help to identify these errors and to refine the models to improve their accuracy. By combining the power of predictive analytics with the wisdom of human judgment, we can make better decisions and achieve better outcomes.
Looking ahead, the convergence of predictive analytics with other emerging technologies, such as the Internet of Things (IoT) and blockchain, promises to unlock even greater potential. The IoT is generating vast amounts of data from sensors and devices embedded in the physical world, providing a rich source of information for predictive models. For example, data from sensors on industrial equipment can be used to predict equipment failures and schedule preventative maintenance. Data from sensors in smart homes can be used to optimize energy consumption and improve home security. By integrating Maplestar with IoT platforms, organizations can gain real-time insights into their operations and make more informed decisions. Blockchain technology can be used to improve the security and transparency of data used in predictive analytics. Blockchain's distributed ledger technology makes it difficult to tamper with data, ensuring that the data used to train predictive models is accurate and reliable. Blockchain can also be used to track the provenance of data, providing transparency into how the data was collected and processed. This can help to build trust in the results of predictive models and to ensure that they are used ethically. By combining Maplestar with IoT and blockchain technologies, we can create a more connected, intelligent, and trustworthy world.
Ultimately, the value of Maplestar lies not just in its technical capabilities, but in its ability to empower people to make better decisions. By providing access to advanced analytics tools and insights, Maplestar can help organizations to improve their performance, to innovate more effectively, and to create more value for their customers. It can also help individuals to make better decisions in their personal lives, such as managing their finances, improving their health, and planning for their future. However, the successful adoption of Maplestar requires more than just technology. It requires a culture of data literacy, a commitment to ethical principles, and a willingness to embrace change. Organizations need to invest in training and education to ensure that their employees have the skills and knowledge needed to effectively use predictive analytics tools. They also need to develop clear guidelines and policies for the ethical use of these technologies. And they need to be prepared to adapt their processes and workflows to take advantage of the insights generated by predictive models. By embracing these changes, organizations can unlock the full potential of Maplestar and create a more data-driven and successful future.
The evolution of Maplestar into a platform that supports truly personalized experiences is also noteworthy. In today's hyper-competitive market, customers expect personalized interactions and offers that are tailored to their individual needs and preferences. Maplestar can help organizations to deliver these personalized experiences by analyzing customer data and identifying patterns in their behavior. For example, an e-commerce retailer could use Maplestar to recommend products that are likely to appeal to a particular customer, based on their past purchases and browsing history. A bank could use it to offer personalized financial advice, based on the customer's income, expenses, and financial goals. A healthcare provider could use it to provide personalized treatment plans, based on the patient's medical history, genetic information, and lifestyle choices. By delivering personalized experiences, organizations can improve customer satisfaction, increase customer loyalty, and drive revenue growth. However, it's important to be mindful of privacy concerns when collecting and using customer data. Organizations need to be transparent about how they are using customer data, and they need to give customers the option to opt out of data collection. They also need to ensure that customer data is stored securely and protected from unauthorized access. By addressing these privacy concerns proactively, organizations can build trust with their customers and create a sustainable model for personalized experiences.
A critical aspect often overlooked is the necessity for continuous model monitoring and retraining when implementing a platform like Maplestar. The world is dynamic; customer behaviors shift, market conditions fluctuate, and new data streams become available. A predictive model trained on data from one point in time will inevitably become less accurate over time as these changes occur. This phenomenon, known as model drift, can lead to inaccurate predictions and poor decision-making. To mitigate this risk, it's essential to continuously monitor the performance of predictive models and to retrain them regularly using the latest data. This ensures that the models remain accurate and relevant. Model monitoring involves tracking key metrics, such as accuracy, precision, and recall, and setting alerts to notify users when the model's performance falls below a certain threshold. When an alert is triggered, the model needs to be retrained using the latest data. The frequency of retraining depends on the rate of change in the underlying data. In some cases, retraining may be required daily, while in other cases it may be sufficient to retrain the model weekly or monthly. Automated model retraining tools can help to streamline this process and to ensure that models are retrained regularly. By continuously monitoring and retraining predictive models, organizations can maintain their accuracy and relevance and avoid the pitfalls of model drift.
In the long run, the true measure of Maplestar's success will be its ability to contribute to a more sustainable and equitable world. As predictive analytics technologies become more pervasive, it's crucial to ensure that they are used to address some of the world's most pressing challenges, such as climate change, poverty, and inequality. Maplestar can be used to optimize resource allocation, to improve the efficiency of supply chains, and to reduce waste. It can also be used to identify communities at risk of environmental disasters and to provide them with timely assistance. Furthermore, Maplestar can be used to promote social justice by identifying and addressing biases in existing systems. For example, it can be used to identify disparities in access to healthcare, education, and employment, and to develop interventions to address these disparities. By using predictive analytics to address these challenges, we can create a more sustainable and equitable future for all. However, it's important to remember that technology is not a panacea. The successful application of Maplestar to these challenges requires a collaborative effort between technologists, policymakers, and community stakeholders. We need to have open and honest conversations about the potential risks and benefits of these technologies, and we need to develop clear guidelines for their use. Only then can we ensure that Maplestar and other predictive analytics tools are used to create a better world.
- Decoding The Megnutt Of Leak Nasas Unusual Measurement
- Unveiling Marianne Bachmeier Honor Murder Amp Shocking Case
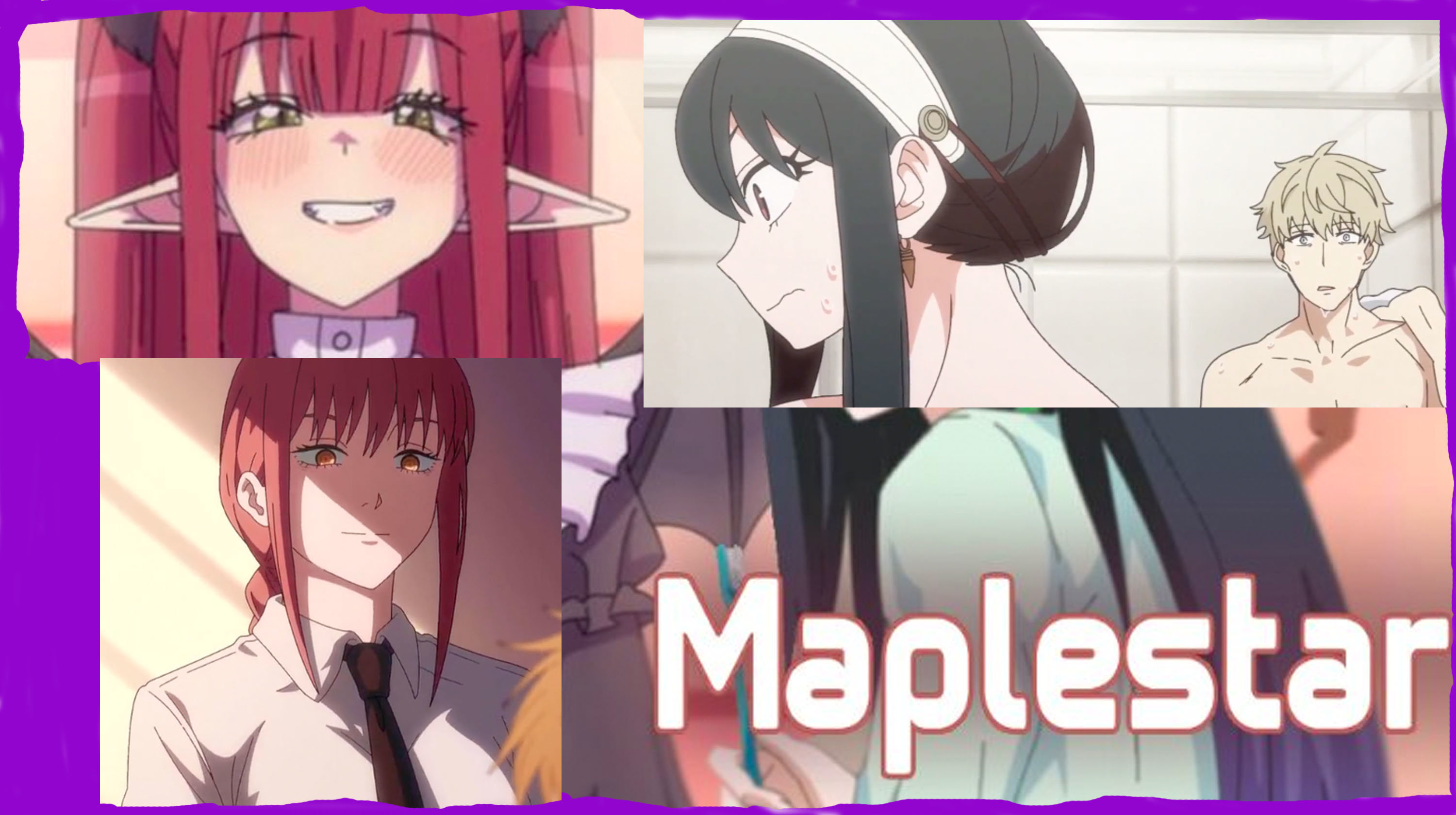
Discovering The Magic Of Maplestar
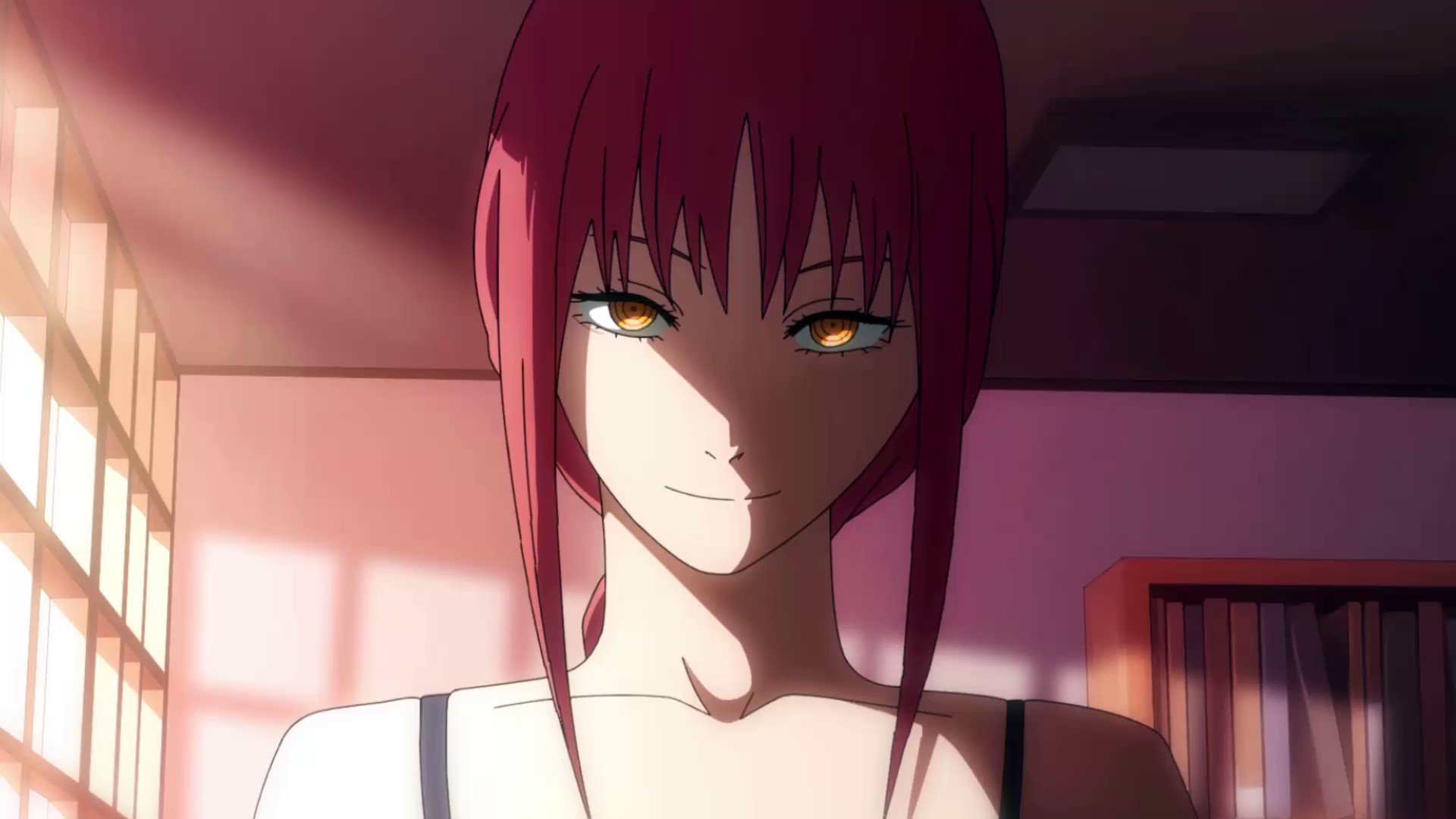
Makima.maplestar ️ Best adult photos at onlynaked.photos
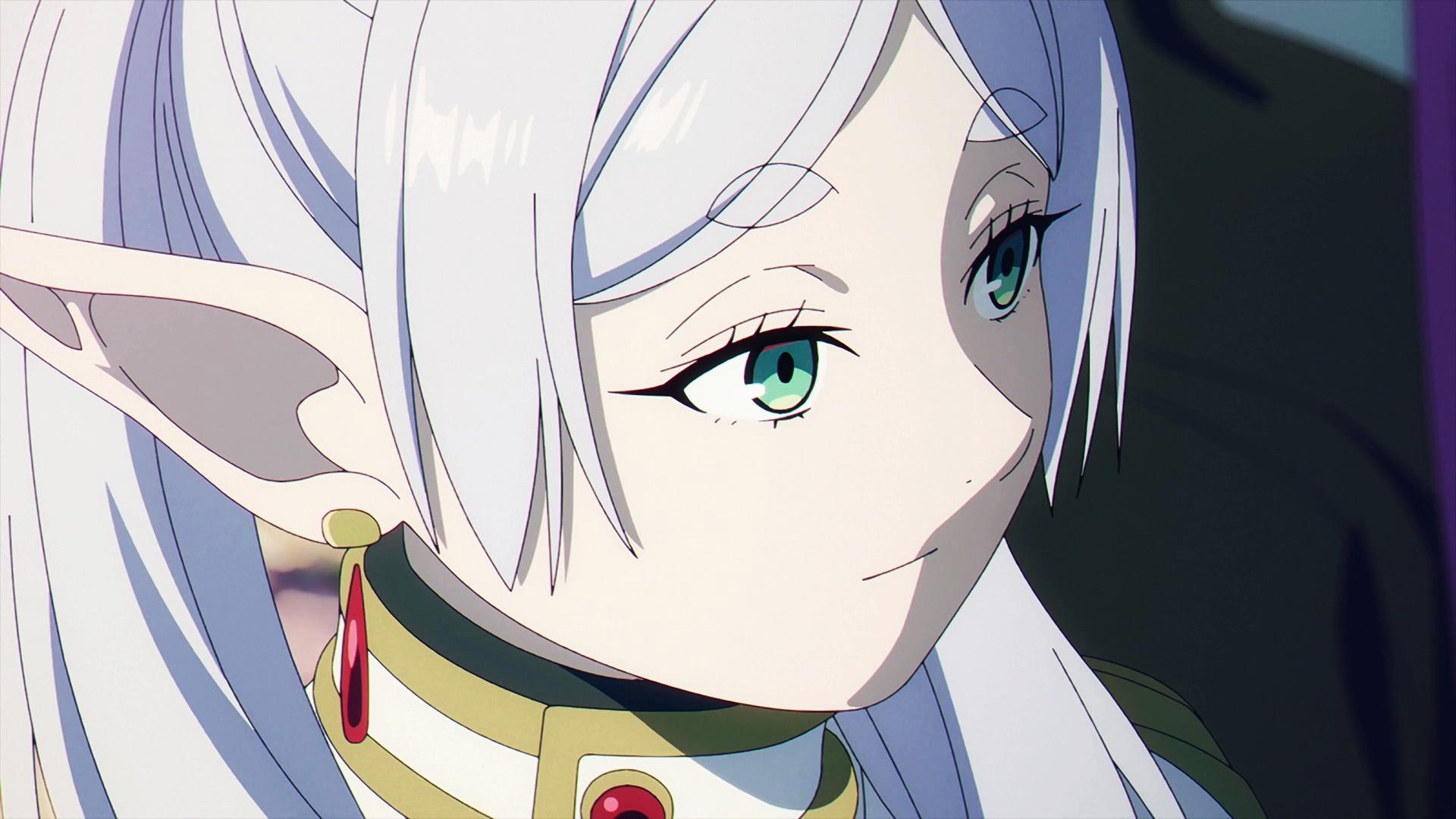
Maplestar The Ultimate Artist for Hentai Animations and